Measuring Bias in the Selection Processes of Political Institutions Restricted; Files Only
Cohen, Elisha (Summer 2022)
Published
Abstract
There are an incredibly wide variety of applications, from elections, to police stops, to hiring that can have bias in the selection process. Across these applications discriminatory processes share a common major inferential challenge: How do we evaluate the selection process when we only observe people who have been selected? For many areas of political science, the people and institutions do not lend themselves to running experiments or randomized controlled trials because it would be unfeasible and/or unethical. Additionally, we often only have data on our units of observation after selection has occurred. To understand these processes, in the following essays I develop credible methodological approaches for non-experimental contexts with selected data and then I apply these methods to evaluate selection into political institutions. The methodological approach I focus on is the outcome test. Outcome tests are a method for detecting bias in selection procedures using data on selected units. In the first chapter, I use a principal stratification approach to establish lower bounds on this bias for general outcomes and weaken the assumptions required for those bounds. In the second chapter on binary outcomes, I derive a lower bound in terms of relative risks and develop a sensitivity analysis to weaken the selection-on-observables assumption. I show that the Knox, Lowe, and Mummolo (2020) bound is sharp for a binary outcome and that the analogous bound for non-binary outcomes is not sharp and provide sharper lower bounds. In the third chapter I apply these techiniques to the electoral context. I illustrate these methods in three ways: 1) with a re-analysis of the data in Anzia and Berry (2011) on the delivery of federal spending by male and female members of Congress, 2) data from the Chicago Police Department to measure gender bias in hiring, and 3) data on U.S. House candidates campaign spending, vote share and federal outlays to measure gender bias in getting elected to Congress.
Table of Contents
1 Overview 1
1.1 Introduction 1
1.2 Chapter Summaries 3
1.2.1 Estimating Bounds on Selection Bias with Outcome Tests 3
1.2.2 Sensitivity Analysis for Outcome Tests with Binary Data 4
1.2.3 Measuring Congressional Gender Bias from Candidate to Representative
4
2 Estimating Bounds on Selection Bias with Outcome Tests 6
2.1 Introduction 6
2.2 Outcome Tests via Principal Stratification 8
2.2.1 Principal Stratification and Post-Selection Outcomes 8
2.2.2 Lower Bound 10
2.2.3 Tightening the Lower Bound 11
2.2.4 Simulated example to demonstrate tightening of the lower bound 14
2.2.5 Including Covariates 14
2.3 Estimating sex-discrimination using legislator effectiveness 15
2.3.1 Including Covariates 17
2.4 Conclusion 19
3 Sensitivity Analysis for Outcome Tests with Binary Data 32
3.1 Introduction: Evaluating Bias in Decision Making Processes 32
3.2 Outcome Test using Potential Outcomes 34
3.2.1 Parameter of Interest 38
3.3 Lower Bound Estimation 39
3.4 Sensitivity Analysis 40
3.4.1 Explaining away the entire effect 43
3.4.2 Comparison covariate 45
3.5 Empirical Application: Women in Policing 47
3.5.1 Women in policing bias 48
3.5.2 Chicago Police Department Data 49
3.5.3 Outcome Test Results on Chicago Police Department Data 50
3.5.4 Sensitivity analysis adjustment 51
3.6 Discussion/Conclusion 54
4 Measuring Congressional Gender Bias from Candidate to Representative 69
4.1 Introduction 69
4.2 Explaining challenges to women’s representation in Congress 72
4.2.1 Descriptive representation of women in Congress 72
4.2.2 Direct discrimination 73
4.2.3 Opportunity structures 74
4.2.4 Candidate emergence 75
4.2.5 Quality Differential 76
4.3 Application 77
4.3.1 Measure of Bias 77
4.4 Data 81
4.4.1 Dichotomizing continuous data 81
4.4.2 Campaign Finance 82
4.4.3 Vote Share 83
4.4.4 Federal Outlays 83
4.5 Analysis and Results 85
4.5.1 Bias in choosing to run 85
4.5.2 Winning the primary 87
4.5.3 Bias in getting elected in the general election 89
4.6 Discussion 100
4.7 Conclusion 101
5 Conclusion 115
Appendix A Estimating Bounds on Selection Bias with Outcome Tests118
A.1 Details on Model Specification 118
A.2 Widows 119
A.3 Matching 119
A.4 Binary Outcome 121
A.5 Strict lower bound 123
A.6 NOC 125
Appendix B Sensitivity Analysis for Outcome Tests with Binary Data127
B.1 Sample Average Definitions 127
B.2 Derivation of π from Equation 3.1 128
B.3 Estimating with a logistic regression 129
Appendix C Measuring Congressional Gender Bias from Candidate to
Representative 137
C.1 Appendix Data Notes 137
C.1.1 Data for Candidate Emergence 137
C.1.2 Winning the primary 139
C.1.3 Winning the general 140
C.1.4 Additional variables 141
C.2 Additional model specifications 142
About this Dissertation
School | |
---|---|
Department | |
Degree | |
Submission | |
Language |
|
Research Field | |
Palavra-chave | |
Committee Chair / Thesis Advisor | |
Committee Members |
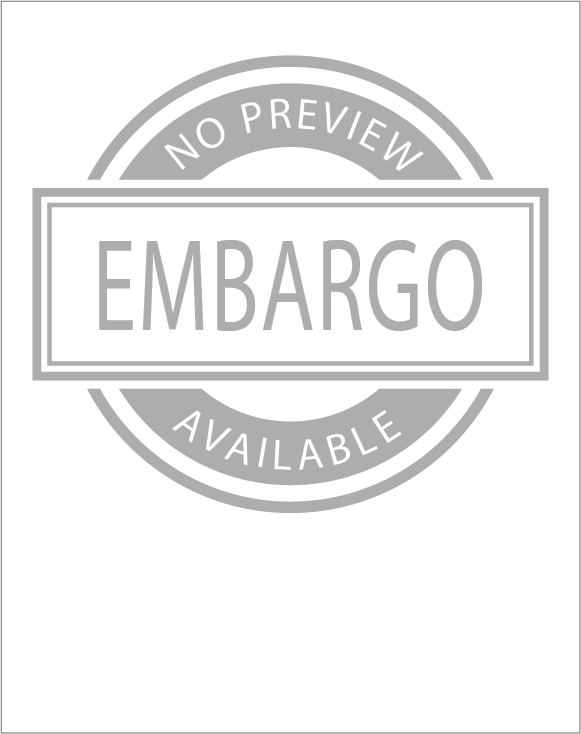
Primary PDF
Thumbnail | Title | Date Uploaded | Actions |
---|---|---|---|
![]() |
File download under embargo until 19 August 2024 | 2022-07-14 09:01:57 -0400 | File download under embargo until 19 August 2024 |
Supplemental Files
Thumbnail | Title | Date Uploaded | Actions |
---|