A Systematic Review of Machine Learning Prediction and Cardiovascular Risk Assessment Público
Trimble, Shawn (Spring 2022)
Abstract
Artificial intelligence (AI) techniques such as machine learning (ML) and deep learning (DL) promise considerable improvements in cardiovascular disease (CVD) prediction. This systematic review and meta-analysis aim to assess and compare the predictive ability of ML algorithms to conventional risk assessment methods in CVDs. Cochrane, Embase, Scopus and Web of Science databases were searched for studies published between January 1, 2012, and January 1, 2022. Studies including the predictive performance of ML models and conventional risk assessment methods were included. Studies without sufficient evaluation data and model validation were excluded. Diagnostic accuracy data was extracted and used to create contingency tables to derive performance metrics of interest: sensitivity, specificity, threshold limits, and areas under the curve (AUC). Studies were included in a meta-analysis, using a bivariate random effects model. The search identified 1688 studies of which 25 studies were included. For the prediction of CVD, ML models had a pooled AUC of 0.88 and conventional risk assessment had a pooled AUC of 0.74. For the prediction of coronary artery disease, ML models had a pooled AUC of 0.83. For the prediction of heart failure, ML models had a pooled AUC of 0.90. For the prediction of stroke, ML models had a pooled AUC of 0.83. Of the 25 studies, 9 studies provided conventional risk assessment comparators. ML models had a pooled AUC of 0.82 compared to conventional risk assessment who had a pooled AUC of 0.78. Insufficient samples sizes for ML models prevented within ML comparisons and analysis of cardiac arrythmias. The predictive ability of ML models is comparable to conventional risk assessment methods. However, heterogeneity among ML models and insufficient data reporting methods within the literature calls into question the clinical applicability of these findings. This review may assist clinicians in assessing the current state of AI and provide insights to how ML models can better translate into the clinical setting.
Table of Contents
Chapter 1: Introduction 1
Section 1.1: Background 1
Section 1.2: Statement of the Problem 3
Section 1.3: Statement of the Purpose 3
Section 1.4: Research Aims 3
Section 1.5: Significance of the Study 3
Chapter 2: Literature Review 4
Section 2.1: Cardiovascular Disease 4
Section 2.1.1: Current Burden 4
Section 2.1.2: Epidemiological, Economic, and Social Impact 5
Section 2.1.3: Risk Assessment 8
Section 2.2: Artificial Intelligence, Machine Learning, Neural Networks, and Deep Learning 9
Section 2.2.1: Artificial Intelligence 9
Section 2.2.2: Machine Learning 10
Section 2.2.3: Deep Learning 12
Section 2.3: Artificial Intelligence Clinical Applications 13
Section 2.3.1: Precision Medicine 13
Section 2.3.2: Clinical Prediction 15
Section 2.3.3: Diagnostic Imaging 16
Section 2.4: AI Concerns and Limitations 17
Section 2.4.1: Data Consent 17
Section 2.4.2: Data Privacy 18
Section 2.4.3: Data Transparency 20
Chapter 3: Methods 21
Section 3.1: Search Strategy 21
Section 3.2: Study Selection 21
Section 3.3: Data Extraction 22
Section 3.4: Statistical Analysis 23
Chapter 4: Results 24
Section 4.1: Study Search 24
Section 4.2: Study Characteristics 25
Section 4.3: Study Results 25
Section 4.3.1: Machine Learning Models and Prediction of Cardiovascular Disease 25
Section 4.3.2: Machine Learning Models and Prediction of Cardiac Arrythmias 27
Section 4.3.3: Machine Learning Models and Prediction of Coronary Artery Disease 27
Section 4.3.4: Machine Learning Algorithms and Prediction of Heart Failure 28
Section 4.3.5: Machine Learning Algorithms and Prediction of Stroke 29
Section 4.3.6: Matched Prediction of Cardiovascular Disease 30
Chapter 5: Discussion 32
Section 5.1: Discussion of Key Findings 32
Section 5.2: Strengths and Weaknesses 34
Section 5.3: Recommendations 35
Section 5.3.1: Quality Assessment Tools 35
Section 5.3.2: Meta-Analysis of Test Performance Gold Standards 36
Section 5.3.3: Clinical Level Contextualization 37
Section 5.4: Conclusion 39
References 41
Appendix 58
Section A-1: PRISMA Checklist 58
Section A-2: Search Strategy 59
Section A-3: Study Characteristics 62
About this Master's Thesis
School | |
---|---|
Department | |
Degree | |
Submission | |
Language |
|
Research Field | |
Palavra-chave | |
Committee Chair / Thesis Advisor |
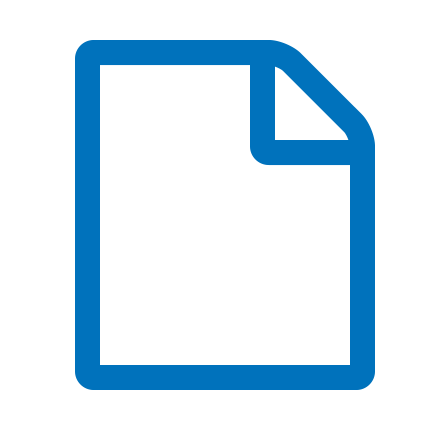
Primary PDF
Thumbnail | Title | Date Uploaded | Actions |
---|---|---|---|
![]() |
A Systematic Review of Machine Learning Prediction and Cardiovascular Risk Assessment () | 2022-04-21 11:07:00 -0400 |
|
Supplemental Files
Thumbnail | Title | Date Uploaded | Actions |
---|