Innovative methods for investigating the genetic architecture of complex human traits Restricted; Files Only
Head, Susan (Spring 2024)
Abstract
Over the past two decades, there has been a rapid increase in the amount of publicly-available genetic datasets necessary to advance gene mapping of complex human traits and diseases. As the amount of genome-wide association (GWAS) data has grown, so has the need for novel statistical methods that aim to not only locate risk regions across the genome, but also shed light on the mechanisms by which these risk loci exert their effect on traits of interest. In this dissertation, we develop and apply innovative statistical methods to help fill these important gaps in such knowledge.
In the first project, we develop a population-based test for parent-of-origin effects (POEs) leveraging GWAS data on multiple phenotypes. A POE exists when maternally- and paternally-transmitted alleles exhibit differential effects on phenotype expression. We show that the presence of a POE at a given locus induces a difference in the covariance structure among multiple phenotypes between homozygotes and heterozygotes. Based on a robust omnibus test for homogeneity of covariance matrices, our method can be applied to normal and non-normal phenotypes and can easily adjust for population stratification and other non-genetic confounders. We evaluate our method through simulation studies and apply it to GWAS data of BMI and two cholesterol phenotypes from the UK Biobank, identifying 338 genome-wide significant variants.
In the second project, we apply a recently proposed transcriptome-wide association study (TWAS) method to publicly available summary statistic GWAS data for breast and ovarian cancer. This Bayesian genome-wide method incorporates both cis- and trans-expression quantitative trait loci (eQTLs). We first train gene expression imputation models using GTEx V8 transcriptomic data separately in breast and ovarian tissue. We then identify genes significantly associated with risk of both cancers and 10 common subtypes of these cancers and investigate the eQTL architecture of these top genes. We show that several novel loci are identified driven primarily by trans-eQTL effects. We replicate several associations using independent GWAS data and expression data in tumor and tumor-adjacent breast tissue from the Cancer Genome Atlas.
In the third project, we expand upon a recent method for TWAS that circumvents the need for individual-level genotype and transcriptomic data. This method leverages summary-level eQTL data and polygenic risk score (PRS) models to impute gene expression in individuals of a given ancestral group. In contrast to ancestrally homogenous populations, recently admixed populations have genomes that are a mosaic of distinct local ancestral (LA) segments, and it is well-known that PRS methods port very poorly across ancestral groups. Motivated by this, we propose a method to perform TWAS with summary-level eQTL data in recently admixed subjects. We compare the imputation accuracy, power, and type I error rate of this LA-aware approach to LA-unaware PRS methods. We apply our method to 29 blood biochemistry phenotypes in two-way African/European admixed individuals in the UK Biobank.
Table of Contents
1 Introduction
1.1 Overview
1.2 Outline of Research
2 Topic 1. POIROT: A powerful test for parent-of-origin effects in unrelated samples leveraging multiple phenotypes
2.1 Introduction
2.2 Methods
2.2.1 Phenotype Model
2.2.2 POIROT Method to Detect POE SNPs
2.2.3 Post-Hoc Test for Interaction Effects
2.2.4 Simulation Study
2.2.5 Application of POIROT to UK Biobank
2.3 Results
2.3.1 Type I Error Rate
2.3.2 Power
2.3.3 Post-Hoc Interaction Test
2.3.4 Applied Data Analysis
2.4 Discussion
2.5 Appendix
2.5.1 Proofs
2.5.2 Tables
2.5.3 Figures
3 Topic 2. Cis- and trans-eQTL TWAS of breast and ovarian cancer identify more than 100 risk associated genes in the BCAC and OCAC consortia
3.1 Introduction
3.2 Materials and Methods
3.2.1 GTEx V8 Training Dataset
3.2.2 Breast Cancer GWAS Summary Data
3.2.3 Ovarian Cancer GWAS Summary Data
3.2.4 Model Training and Association Test
3.2.5 Validation Analyses
3.3 Results
3.3.1 Fitted GReX Models and eQTL Architecture
3.3.2 Breast Cancer TWAS
3.3.3 Ovarian Cancer TWAS
3.4 Discussion
3.5 Web Resources
3.6 Appendix
3.6.1 Tables
3.6.2 Figures
4 Topic 3. Enhanced transcriptome-wide association analyses in admixed samples using eQTL summary data
4.1 Introduction
4.2 Materials and Methods
4.2.1 Overview
4.2.2 Modeling Expression in Admixed Individuals
4.2.3 Stage I Reference Expression Model Training via OTTERS
4.2.4 Stage II Imputing Expression in Admixed Individuals
4.2.5 Stage II Gene-Trait Association Test
4.2.6 Simulations
4.2.7 Applied Analysis
4.3 Results
4.3.1 Expression Imputation Accuracy
4.3.2 Type I Error Rate
4.3.3 Power
4.3.4 Applied Data Analysis
4.4 Discussion
4.5 Appendix
4.5.1 Tables
4.5.2 Figures
4.5.3 Proofs
5 Future Work
Bibliography
About this Dissertation
School | |
---|---|
Department | |
Degree | |
Submission | |
Language |
|
Research Field | |
Mot-clé | |
Committee Chair / Thesis Advisor | |
Committee Members |
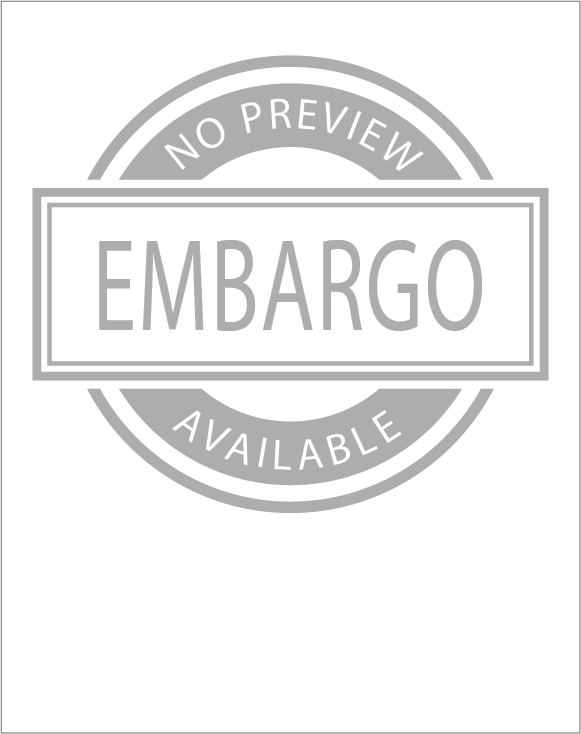
Primary PDF
Thumbnail | Title | Date Uploaded | Actions |
---|---|---|---|
![]() |
File download under embargo until 22 November 2024 | 2024-04-22 08:30:53 -0400 | File download under embargo until 22 November 2024 |
Supplemental Files
Thumbnail | Title | Date Uploaded | Actions |
---|