Relaxed Conditional Bootstrap Inference for Sparse Data Under a Rectangular Array Embedding Restricted; Files Only
Aiyedipe, Samuel (Spring 2022)
Abstract
The presence of numerous nuisance parameters complicates statistical inference, often creating complicated tradeoffs between unbiasedness and precision. In a rectangular array asymptotic setting, complications arise with naive use of the unconditional maximum likelihood approach. Fitting of the nuisance parameters can result in high bias in the parameters of interest (Li et al. 2003; Sartori 2003; Hahn and Newey 2004). In the special case of an exponential family with a complete sufficient statistic for the nuisance parameters, wholly conditional inference is often efficient (Liang 1984). However, if the minimal sufficient statistics for the nuisance parameters are not complete, wholly conditional inference might sacrifice efficiency for the sake of reducing bias. In such situations, a relaxed conditional likelihood has been proposed to increase efficiency (Hanfelt and Wang 2014). As the most efficient version of the relaxation index requires difficult calculations, these authors instead recommended the use of a relatively simple approximation to the optimal relaxation index that works well provided the data are not too sparse.
In this research, we propose to fully develop the relaxed conditional likelihood approach under a rectangular array asymptotic setting. Our specific aims are the following. First, we propose a bootstrap estimator of the optimal relaxation index and examine its properties using Monte-Carlo simulation. Next, we extend the work of Hanfelt and Wang (2014) by proposing relaxed conditional likelihood ratio tests and relaxed conditional score tests. We develop the asymptotic theory for these tests and demonstrate in a Monte-Carlo simulation study that our approach yields valid performance in medium-sized samples in terms of size and power under local alternatives.
Finally, in the more challenging situation when the minimum sufficient statistics for the nuisance parameters are functions of the parameter of interest and incomplete, we develop a relaxed version of Lindsay’s (1982) conditional score function approach. We propose two large-sample tests under a rectangular asymptotic setting. We show in a simulation study that our method yields improved performance in medium-sized samples.
Table of Contents
1 Introduction 1
1.1 Overview . . . . . . . . . . . . . . . . . . . . . . . . . . . . . . . . . . 2
1.2 Scope of Dissertation . . . . . . . . . . . . . . . . . . . . . . . . . . . 3
1.3 Statistical Inference in Presence of Numerous Nuisance parameters
when the Minimum Sufficient Statistic for Nuisance Parameter is Functionally
Independent on the Parameter of Interest. . . . . . . . . . . . 4
1.3.1 Profile Likelihood . . . . . . . . . . . . . . . . . . . . . . . . . 4
1.3.2 Conditional Likelihood . . . . . . . . . . . . . . . . . . . . . . 5
1.3.3 Relaxed Conditional Likelihood . . . . . . . . . . . . . . . . . 5
1.4 Statistical Inference in Presence of Numerous Nuisance Parameters
when the Sufficient Statistic for Nuisance Parameter is Functionally
Dependent on the Parameter of Interest. . . . . . . . . . . . . . . . . 7
1.4.1 Lindsay’s Conditional Score Function . . . . . . . . . . . . . . 7
1.4.2 Relaxed Conditional Score Function . . . . . . . . . . . . . . . 7
2 OPTIMAL RELAXED CONDITIONAL LIKELIHOOD INFERENCE 9
2.1 Introduction . . . . . . . . . . . . . . . . . . . . . . . . . . . . . . . . 10
2.2 Relaxed Conditional Likelihood . . . . . . . . . . . . . . . . . . . . . 10
2.2.1 Summary of the asymptotic properties of the Relaxed Conditional
Likelihood . . . . . . . . . . . . . . . . . . . . . . . . . 12
2.2.2 Proposed estimator of the optimal index . . . . . . . . . . . . 13
2.2.3 Inference . . . . . . . . . . . . . . . . . . . . . . . . . . . . . . 14
2.3 Alternative optimality criteria . . . . . . . . . . . . . . . . . . . . . . 15
2.4 Numerical Examples . . . . . . . . . . . . . . . . . . . . . . . . . . . 15
2.5 Discussion . . . . . . . . . . . . . . . . . . . . . . . . . . . . . . . . . 16
3 OPTIMAL RELAXED CONDITIONAL SCORE FUNCTION 21
3.1 Introduction . . . . . . . . . . . . . . . . . . . . . . . . . . . . . . . . 22
3.2 Relaxed Conditional Score Function . . . . . . . . . . . . . . . . . . . 23
3.2.1 Summary of the asymptotic properties of the Relaxed Conditional
Likelihood . . . . . . . . . . . . . . . . . . . . . . . . . 23
3.2.2 Optimal relaxation index . . . . . . . . . . . . . . . . . . . . . 24
3.2.3 Proposed estimator of the optimal relaxation index . . . . . . 25
3.2.4 Inference . . . . . . . . . . . . . . . . . . . . . . . . . . . . . . 25
3.3 Alternative optimality criteria . . . . . . . . . . . . . . . . . . . . . . 26
3.4 Numerical Examples . . . . . . . . . . . . . . . . . . . . . . . . . . . 26
3.5 Discussion . . . . . . . . . . . . . . . . . . . . . . . . . . . . . . . . . 27
4 SUMMARY 30
A Appendix for Chapter 2 32
A.1 Proof of Theorem 2.1 . . . . . . . . . . . . . . . . . . . . . . . . . . . 32
B Appendix for Chapter 3 45
B.1 Proof of Theorem 3.1 . . . . . . . . . . . . . . . . . . . . . . . . . . . 45
B.2 Proof of Theorem 3.2 . . . . . . . . . . . . . . . . . . . . . . . . . . . 47
Bibliography 53
About this Dissertation
School | |
---|---|
Department | |
Degree | |
Submission | |
Language |
|
Research Field | |
Stichwort | |
Committee Chair / Thesis Advisor | |
Committee Members |
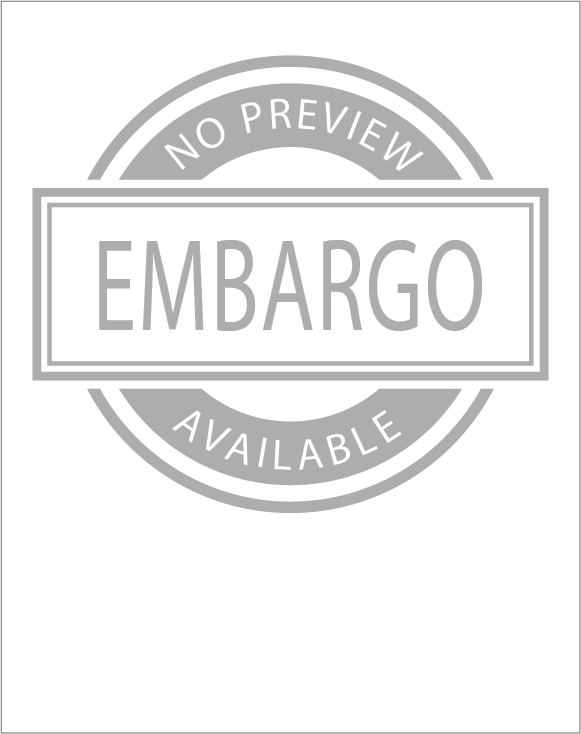
Primary PDF
Thumbnail | Title | Date Uploaded | Actions |
---|---|---|---|
![]() |
File download under embargo until 26 May 2028 | 2022-04-14 17:30:46 -0400 | File download under embargo until 26 May 2028 |
Supplemental Files
Thumbnail | Title | Date Uploaded | Actions |
---|