Artificial Intelligence and the Future of Work: Empirical Studies in Occupational Outlook and Firm Performance Restricted; Files Only
Yeo, Jinsoo (Summer 2023)
Published
Abstract
This dissertation focuses on two essential aspects of Artificial Intelligence (AI): its impact on occupations and firm performance. As AI continues to develop at exponential rates, scholarly work in these areas holds timely and significant relevance. First, evidence from past technological development suggests that AI’s disruption is likely to be skill-biased. Considering the wage and employment polarization that have followed digital disruptions for the last few decades, the world stands on the brink of an even more profound and far-reaching disruption. As occupations connect employment and wages, analyzing AI-enabled occupational changes is pivotal to ensure that policy and regulatory controls are in place to support development and mitigate social disruption. Second, as firms are the primary drivers of business processes, the latest empirical evidence on firm performance provides subtle insights into the efficiency, underlying mechanisms, and strategies for utilizing AI technology.
Regarding occupational impact, this dissertation seeks to identify which jobs are more amenable/resistant towards AI, and more importantly, why. Using a multifaceted approach, I revisit extant studies by leveraging the comprehensive O*NET dataset. Utilizing this rich data source as a feature vector, I employ a black-box machine learning model, capturing the intricate relationship between AI and various occupations. To generate a model that can potentially explain its underlying mechanisms, I approximate the predicted indices, with an explainable model, a decision tree. This approach allows nuanced and systematic interpretation. Notably, “Social Perceptiveness” is consistently identified as the root node, indicating that it is the single most informative factor that explains occupational AI exposure. Regarding firm performance, this dissertation analyzes the association between AI skill investment and firm performance, and whether this relationship deviates between AI-using and AI-inventing firms. Using a dynamic panel model, I show that firms investing in AI experience market value gain but not in productivity. Subsequent analysis reveals that market value is significantly associated with AI-using firms but not AI-inventing firms. This suggests that in the short run, the market tends to reward AI usage, emphasizing quicker returns over longer and more uncertain AI invention.
Table of Contents
1. Introduction: 1
1.1. Research Problem, Context, and Dissertation Approach: 1
1.2. Summary of Essay1: AI and the Future of Work: A Supervised Learning Approach to Explain Occupational Exposure: 4
1.3. Summary of Essay2: AI and Firm Performance : A Comparative Study of AI-Using and AI-Inventing Firms: 6
2. AI and the Future of Work: A Supervised Learning Approach to Explain Occupational Exposure: 9
2.1. Introduction: 9
2.2. Literature Review: 14
2.3. Construction of the AI Amenability Measure: 31
2.4. Interpretation of the AI Amenability Measure: 42
2.5. Conclusion: 52
3. Artificial Intelligence and Firm Performance: A Comparative Study of AI-Using and AI-Inventing Firms: 53
3.1. Introduction: 53
3.2. Data: 57
3.3. Methodology and Descriptive Evidence: 61
3.4. AI Investment and Firm Performance: 68
3.4.1 Firm Productivity: 68
3.4.2 Firm Market Value: 69
3.4.3 Robustness Check: 73
3.4.4 Differential Impact between AI-using firms and AI-inventing firms: 79
3.5. Conclusion: 82
4. Conclusion: 84
5. Appendix A: 86
6. Appendix B: 91
About this Dissertation
School | |
---|---|
Department | |
Subfield / Discipline | |
Degree | |
Submission | |
Language |
|
Research Field | |
Keyword | |
Committee Chair / Thesis Advisor | |
Committee Members |
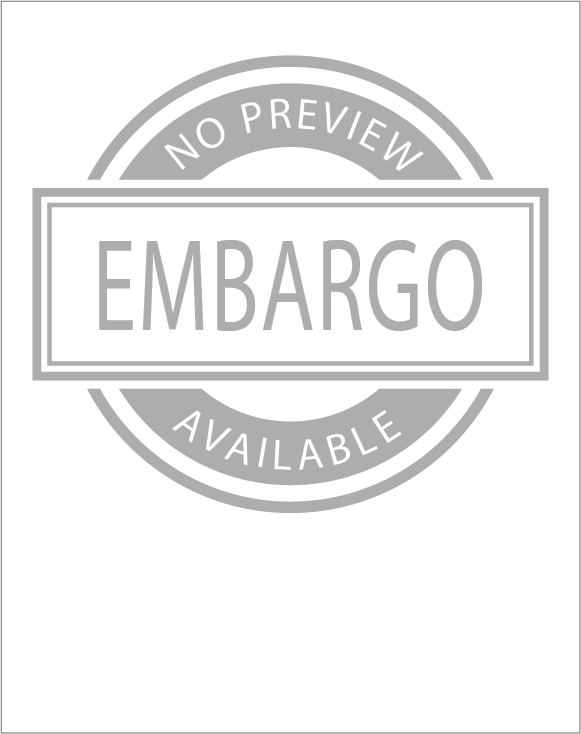
Primary PDF
Thumbnail | Title | Date Uploaded | Actions |
---|---|---|---|
![]() |
File download under embargo until 16 August 2029 | 2023-08-14 18:13:02 -0400 | File download under embargo until 16 August 2029 |
Supplemental Files
Thumbnail | Title | Date Uploaded | Actions |
---|