Improvements in statistical machine learning for current problems in public health Restricted; Files & ToC
Wu, Ziyue (Spring 2022)
Abstract
In this dissertation, we propose methods to deal with current problems in public health research that arises in two areas: studies of healthcare expenditures and of vaccine efficacy.
In topic 1, we propose a two-stage ensemble machine learning approach to improve the prediction of future health care expenditures. The method uses a two-part model to separately estimate the probability of having any healthcare expenditure and the mean amount of healthcare expenditure conditional on having healthcare expenditure. These two estimates are combined to form an ensemble model that provides predictions of expenditures. The method can flexibly incorporate a range of individual algorithms for each stage of estimation, including both regression-based and machine learning algorithms. Extensive simulations and two real data applications suggest distinct improvements in cost estimation of the proposed two-stage super learner compared with the standard one-stage super learner and individual algorithms.
In topic 2, we propose further improvements for the prediction of healthcare expenditures. Here, we propose ensemble models based on the Huber loss function that combines the typical squared error loss with the absolute loss to down-weight the influence of outliers. We demonstrate that our proposed Huber loss-based super learner provides a theoretically optimal way of model selection/ensembling in terms of optimizing the Huber risk. We also show that our approach provides finite sample benefits when optimizing based on mean squared error is the ultimate goal. This latter property is demonstrated via application to semiparametric cost prediction and causal effect estimation.
In topic 3, we propose a new methodology for vaccine sieve analysis in the existence of missing pathogen information. Our proposed method accounts specifically for informative missingness due to low viral load and allows for covariate adjustment through nonparametric modeling of key quantities including a time-varying hazard function, a probability function for missing pathogen strain, and a time-varying distribution of the pathogen strains of interest. We derive results that indicate how to perform point estimates and statistical inferences using our estimators. Realistic simulations show reduced bias and efficiency relative to standard nonparametric competing risks estimators.
Table of Contents
This table of contents is under embargo until 26 May 2028
About this Dissertation
School | |
---|---|
Department | |
Degree | |
Submission | |
Language |
|
Research Field | |
Keyword | |
Committee Chair / Thesis Advisor | |
Committee Members |
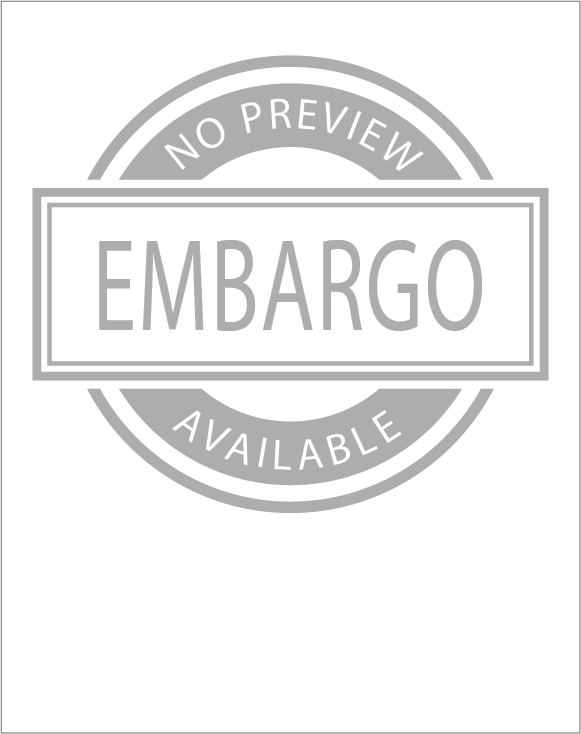
Primary PDF
Thumbnail | Title | Date Uploaded | Actions |
---|---|---|---|
![]() |
File download under embargo until 26 May 2028 | 2022-04-13 14:40:14 -0400 | File download under embargo until 26 May 2028 |
Supplemental Files
Thumbnail | Title | Date Uploaded | Actions |
---|